40 nlnl negative learning for noisy labels
《NLNL: Negative Learning for Noisy Labels》论文解读 - 知乎 对于一个图像分类任务,传统的学习策略PL (Positive Learning)利用图像正确的标签:"input image belongs to this label"。. 但在噪声情况下,PL会提供错误的信息,随着训练的进行会逐渐拟合噪声标签从而降低模型性能。. 因此,作者提出了NL (Negative Learning):"input image does not belong to this complementary label"。. Complementary label 的意思是当前训练图片不属于该标签。. 举个例子,一张狗的图片 ... Nlnl Negative Learning For Noisy Labels - Python Repo Nlnl: Negative Learning For Noisy Labels. Created 02 May, 2020 Issue #4 User Yw981. Hello ,I'm very interested in your work and trying to reproduce your results.
NLNL-Negative-Learning-for-Noisy-Labels/main_NL.py at master ... - GitHub NLNL: Negative Learning for Noisy Labels. Contribute to ydkim1293/NLNL-Negative-Learning-for-Noisy-Labels development by creating an account on GitHub.

Nlnl negative learning for noisy labels
NLNL: Negative Learning for Noisy Labels | DeepAI 08/19/19 - Convolutional Neural Networks (CNNs) provide excellent performance when used for image classification. The classical method of tra... ICCV 2019 Open Access Repository However, if inaccurate labels, or noisy labels, exist, training with PL will provide wrong information, thus severely degrading performance. To address this issue, we start with an indirect learning method called Negative Learning (NL), in which the CNNs are trained using a complementary label as in "input image does not belong to this complementary label." Kim_NLNL_Negative_Learning_for_Noisy_Labels_ICCV_2019_paper.pdf View Kim_NLNL_Negative_Learning_for_Noisy_Labels_ICCV_2019_paper.pdf from CS 559 at Stevens Institute Of Technology. NLNL: Negative Learning for Noisy Labels Youngdong Kim Junho Yim Juseung Yun Junmo. Study Resources. Main Menu; by School; by Literature Title; by Subject; Textbook Solutions Expert Tutors Earn.
Nlnl negative learning for noisy labels. 【噪声损失】合集及对应代码 - 知乎 NLNL (Negative Learning for Noisy Labels) 这篇展开起来稍微有点复杂,主要是为了防止带噪声的数据产生过拟合问题。 首先,先定义了随机选取的补充标签: Research Code for NLNL: Negative Learning for Noisy Labels However, if inaccurate labels, or noisy labels, exist, training with PL will provide wrong information, thus severely degrading performance. To address this issue, we start with an indirect learning method called Negative Learning (NL), in which the CNNs are trained using a complementary label as in "input image does not belong to this ... NLNL: Negative Learning for Noisy Labels - CORE Reader NLNL: Negative Learning for Noisy Labels - CORE Reader PDF NLNL: Negative Learning for Noisy Labels - CVF Open Access called Selective Negative Learning and Positive Learning (SelNLPL), which demonstrates excellent performance for filteringnoisydatafromtrainingdata(Section3.4). Finally, semi-supervised learning is performed for noisy data clas-sification, utilizing the filtering ability of SelNLPL (Sec-tion 3.5). 3.1. Negative Learning
[PDF] NLNL: Negative Learning for Noisy Labels | Semantic Scholar A novel improvement of NLNL is proposed, named Joint Negative and Positive Learning (JNPL), that unifies the filtering pipeline into a single stage, allowing greater ease of practical use compared to NLNL. 5 Highly Influenced PDF View 5 excerpts, cites methods Decoupling Representation and Classifier for Noisy Label Learning Hui Zhang, Quanming Yao [1908.07387v1] NLNL: Negative Learning for Noisy Labels However, if inaccurate labels, or noisy labels, exist, training with PL will provide wrong information, thus severely degrading performance. To address this issue, we start with an indirect learning method called Negative Learning (NL), in which the CNNs are trained using a complementary label as in "input image does not belong to this complementary label." NLNL: Negative Learning for Noisy Labels | Papers With Code However, if inaccurate labels, or noisy labels, exist, training with PL will provide wrong information, thus severely degrading performance. To address this issue, we start with an indirect learning method called Negative Learning (NL), in which the CNNs are trained using a complementary label as in "input image does not belong to this complementary label." PDF Negative Learning for Noisy Labels - UCF CRCV Label Correction Correct Directly Re-Weight Backwards Loss Correction Forward Loss Correction Sample Pruning Suggested Solution - Negative Learning Proposed Solution Utilizing the proposed NL Selective Negative Learning and Positive Learning (SelNLPL) for filtering Semi-supervised learning Architecture
[오늘의 절대식] NLNL: Negative Learning for Noisy Labels [논문 DeepL 번역 ... To address this issue, we start with an indirect learning method called Negative Learning (NL), in which the CNNs are trained using a complementary label as in "input image does not belong to this complementary label."Because the chances of selecting a true label as a complementary label are low, NL decreases the risk of providing incorrect ... Joint Negative and Positive Learning for Noisy Labels 従来手法 4 正解以外のラベルを与える負の学習を提案 Negative learning for noisy labels (NLNL)*について 負の学習 (Negative Learning:NL) と呼ばれる間接的な学習方法 真のラベルを選択することが難しい場合,真以外をラベルとして学習す ることでNoisy Labelsのデータを ... Joint Negative and Positive Learning for Noisy Labels - DeepAI NL [kim2019nlnl] is an indirect learning method for training CNNs with noisy data. Instead of using given labels, it chooses random complementary label ¯ ¯y and train CNNs as in "input image does not belong to this complementary label." The loss function following this definition is as below, along with the classic PL loss function for comparison: NLNL: Negative Learning for Noisy Labels | Request PDF Because the chances of selecting a true label as a complementary label are low, NL decreases the risk of providing incorrect information. Furthermore, to improve convergence, we extend our method...
Joint Negative and Positive Learning for Noisy Labels NLNL further employs a three-stage pipeline to improve convergence. As a result, filtering noisy data through the NLNL pipeline is cumbersome, increasing the training cost. In this study, we...
ydkim1293/NLNL-Negative-Learning-for-Noisy-Labels - GitHub NLNL-Negative-Learning-for-Noisy-Labels. Pytorch implementation for paper NLNL: Negative Learning for Noisy Labels, ICCV 2019. Paper: . Requirements. python3; pytorch; matplotlib; Generating noisy data
Εκπαίδευση Νευρωνικών Δικτύων Παρουσία Θορύβου Ετικετών with sparse and noisy labels, compared to using either of these approaches alone. Keywords. Artificial intelligence, Machine learning, Deep learning, ...78 σελίδες
NLNL: Negative Learning for Noisy Labels - NASA/ADS Convolutional Neural Networks (CNNs) provide excellent performance when used for image classification. The classical method of training CNNs is by labeling images in a supervised manner as in "input image belongs to this label" (Positive Learning; PL), which is a fast and accurate method if the labels are assigned correctly to all images. However, if inaccurate labels, or noisy labels, exist ...
GitHub - ydkim1293/NLNL-Negative-Learning-for-Noisy-Labels: NLNL: Negative Learning for Noisy Labels
NLNL: Negative Learning for Noisy Labels The classical method of training CNNs is by labeling images in a supervised manner as in Convolutional Neural Networks (CNNs) provide excellent performance when used for image classification. IEEE.org
NLNL: Negative Learning for Noisy Labels - Semantic Scholar Figure 5: Pseudo labeling for semi-supervised learning. (a): Division of training data into either clean or noisy data with CNN trained with SelNLPL. (b): Training initialized CNN with clean data from (a), then noisy datas label is updated following the output of CNN trained with clean data. (c): Clean data and label-updated noisy data are both used for training initialized CNN in the final step.
NLNL: Negative Learning for Noisy Labels论文解读 NLNL: Negative Learning for Noisy Labels论文解读 Posted by ivan on 2021-03-22 16:04:11
NLNL-Negative-Learning-for-Noisy-Labels/main_NL.py at master · ydkim1293/NLNL-Negative-Learning ...
Joint Negative and Positive Learning for Noisy Labels Training of Convolutional Neural Networks (CNNs) with data with noisy labels is known to be a challenge. Based on the fact that directly providing the label to the data (Positive Learning; PL) has a risk of allowing CNNs to memorize the contaminated labels for the case of noisy data, the indirect learning approach that uses complementary labels (Negative Learning for Noisy Labels; NLNL) has ...
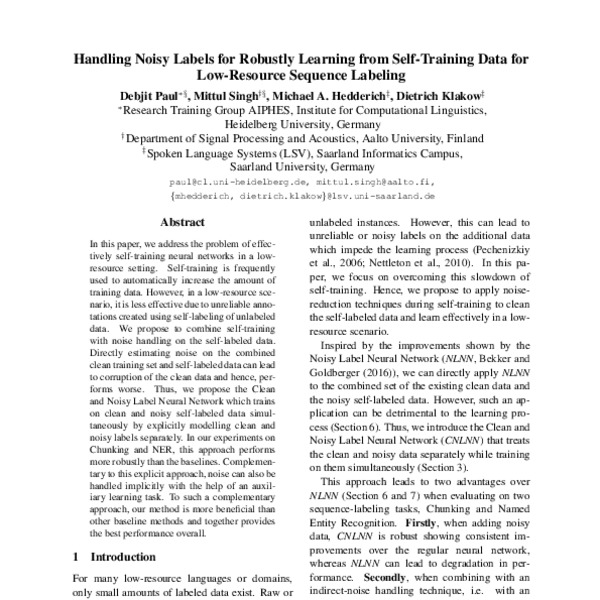
Handling Noisy Labels for Robustly Learning from Self-Training Data for Low-Resource Sequence ...
噪声标签的负训练:ICCV2019论文解析 - 吴建明wujianming - 博客园 . 摘要. 卷积神经网络(CNN)在用于图像分类时具有优异的性能。. 经典的CNNs训练方法是以有监督的方式标记图像,如"输入图像属于此标签"(正学习;PL),如果标签被正确分配给所有图像,这是一种快速而准确的方法。. 然而,如果存在不准确的标签或噪声标签,使用PL进行的培训将 ...
Post a Comment for "40 nlnl negative learning for noisy labels"